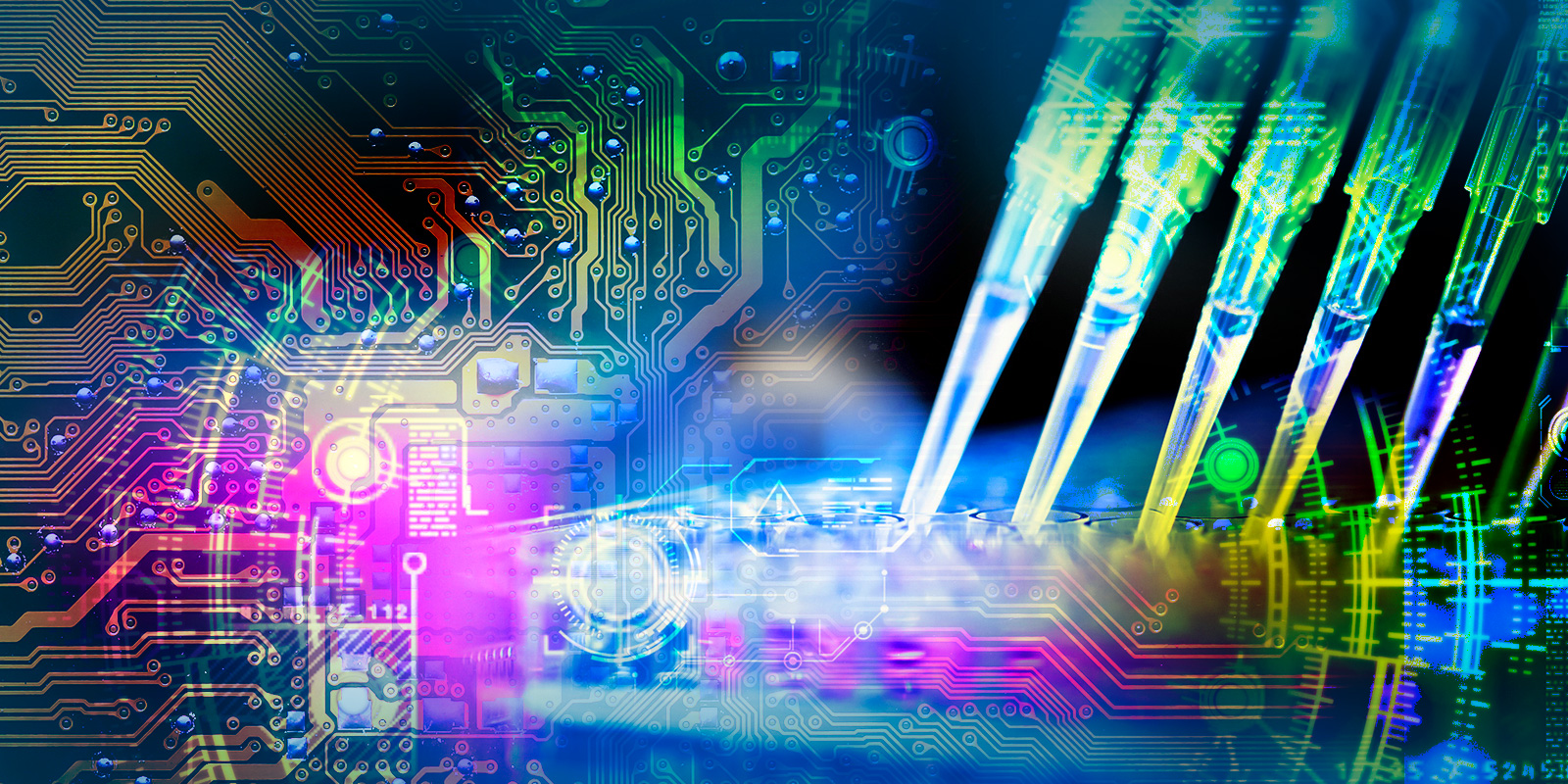
Artificial Intelligence and the Biopharmaceutical Industry: What's Next?
In Short
The Situation: Artificial intelligence ("AI") is emerging as a key driver of innovation in the biopharmaceutical industry, which uses AI in research and development ("R&D") to analyze big data.
The Result: AI is already transforming the R&D process in the biopharmaceutical industry, and that disruption will only increase as AI capabilities expand. At the same time, AI's disruption of traditional concepts of intellectual property ("IP") law will have a significant impact on the biopharmaceutical industry.
Looking Ahead: The United States Patent and Trademark Office ("USPTO") recently sought public comment on a variety of fundamental questions related to AI, including asking whether new forms of IP protection are needed to protect AI-related innovations. The biopharmaceutical industry in the United States should continue to monitor legal developments as they unfold in the USPTO, Congress, and the courts.
Introduction
Leading biopharmaceutical companies, startups, and scientists are integrating AI into R&D in an effort to analyze enormous sets of data, identify patterns, and generate algorithms to explain them. AI is increasingly capable of predictive analytics and problem solving, as well as discovering and "inventing" new innovations without human input [see "When AI Invents: Two Applications Test U.S. Patent Law"; see also "When Innovation Invents: Artificial Intelligence Issues at the U.S. Patent and Trademark Office"; see also "When Innovation Creates: Additional Developments in Artificial Intelligence at the U.S. Patent and Trademark Office"]. While AI has the potential to transform the R&D process in the biopharmaceutical industry, its clinical value has not yet been realized, in part because of a lack of a clear understanding of how to quantify benefit or ensure patient safety. What is certain is that AI has disrupted traditional concepts of IP law, leading to more open questions than clear answers.
Anticipating the AI Impact on Biopharmaceutical R&D
The emerging market for AI in drug development, valued at $700 million in 2018, is predicted to increase to more than $5 billion in 2024. According to one estimate, developing a new drug that gains marketing approval is estimated to cost $2.6 billion and can take 10-20 years[DiMasi, A., Grabowski, Henry G., Hansen, Ronald W. Innovation in the pharmaceutical industry: New estimates of R&D costs. Journal of Health Economics, Volume 47, May 2016, Pages 20-33]. Meanwhile, the clinical trial success rate for new drugs hovers around 10% [Wong, C.H, and Siah, K.W. Estimation of clinical trial success rates and related parameters, Biostatistics. 2019 Apr; 20(2): 273–286].
The use of AI in drug development is particularly promising because it can potentially accelerate every stage of the R&D process while significantly reducing costs. For example, AI is being used in the biopharma industry to identify new candidate drugs, which are then screened, validated, and optimized by AI-driven, integrated structural, target, and pathway-based data analysis. The generation of predictive in silico profiles of candidate drugs can reduce the time and cost of preclinical decision-making while increasing the likelihood of success for compounds selected for clinical trials. Further, the design of clinical trials to test novel drugs can benefit from predictive analytics, which can analyze clinical and genetic information to identify appropriate patient populations for trial inclusion to improve treatment outcomes.
Diagnostics is another area that is highly amenable to AI, as AI-based analytics can sift through massive sets of patient data to identify differences between healthy and unhealthy patients. For example, AI-based visual pattern recognition can be applied to the diagnosis of patients in biomedical imaging, including x-ray, radiography, computed tomography ("CT"), magnetic resonance imaging ("MRI"), mammography, and ultrasound. In fact, machine learning techniques (including deep learning) are currently 5–10% more accurate than the average physician, a gap that is expected to widen [Yun Liu et al., Detecting Cancer Metastases on Gigapixel Pathology Images ARXIV:1703.02442 [CS] (2017), stating "[a]t 8 false positives per image, we detect 92.4% of the tumors, relative to 82.7% by the previous best automated approach. For comparison, a human pathologist attempting exhaustive search achieved 73.2% sensitivity"]. When applied to genomic datasets, AI algorithms are able to identify mutations with a significantly lower error rate, flagging them as pathogenic while revealing crucial information about the molecular pathways involved in a given cancer.
AI technologies can be successfully applied to any massive, well-annotated data set, such as those accumulated in any of the "omics" fields, such as genomics, proteomics, transcriptomics, and metabolomics. In particular, by integrating patient health data, including genetic, epigenetic, and medical records, AI can yield significant advances in personalized medicine, optimizing treatment decision-making for each individual and predicting treatment responses to candidate drugs.
AI's Disruption of Traditional IP Concepts
As noted above, the dialogue on AI's impact on fundamental IP concepts is unsettled—and evolving. For example, there are open questions as to whether and to what extent existing IP laws are adequate to protect innovations that are invented or created by AI. In the context of patents, AI may well impact the definition of a "person" of ordinary skill in the art, predictability, inventorship, and subject matter eligibility. The USPTO has sought public comment on some of these questions and, more generally, the impact of AI technologies on IP law and policy. On August 27, 2019, the USPTO published questions related to the impact of AI inventions on patent law and policy, including: (i) whether current laws and regulations need to be revised to address AI's contribution to the conception of an invention and AI's potential ownership of an invention; (ii) whether there any patent eligibility considerations unique to AI inventions; (iii) whether AI impact the level of a person of ordinary skill in the art; and (iv) whether the disclosure rules (enablement, specification, etc.) need to be altered for AI-related patent applications. On October 17, 2019, the USPTO issued new guidance to address the "mental steps" challenge to patent eligibility of AI inventions, stating that "[c]laims do not recite a mental process when they do not contain limitations that can practically be performed in the human mind." On October 30, 2019, the USPTO extended the inquiry to copyright, trade secret, trademark, and other IP rights impacted by AI [see also "When AI Invents: Two Applications Test U.S. Patent Law"; see also "When Innovation Invents: Artificial Intelligence Issues at the U.S. Patent and Trademark Office"; see also "When Innovation Creates: Additional Developments in Artificial Intelligence at the U.S. Patent and Trademark Office"]. Addressed briefly below are some of the more significant IP considerations for the biopharmaceutical industry.
Important IP Considerations for the Biopharmaceutical Industry
1. What is patentable?
Pharmaceutical companies have traditionally considered patents the strongest method of protecting innovation, and there has been a significant increase in the number of AI-related worldwide patent filings. However, due in large part to the U.S. Supreme Court's decision in Alice, which held that a computerized method was an unpatentable "abstract idea" [Alice Corp. v. CLS Bank International, 573 U.S. 208 (2014)], software-related patent applications and issued patents have been increasingly attacked.
While what is considered an "abstract idea" and thus unpatentable is not always clear, courts have found that an "abstract idea" includes any invention that mimics a human's "mental steps" and "can be performed in the human mind, or by a human using a pen and paper" [CyberSource Corp. v. Retail Decisions, Inc., 654 F.3d 1366 (Fed. Cir. 2011)]. Indeed, one court held that "just because a computer can make calculations more quickly than a human does not render a method patent eligible," even if it would be physically impossible for a human to do so [Purepredictive, Inc. v. H20.AI, Inc., No. 17-cv-03049, at *14 (N.D. Cal. Aug. 29, 2017)]. An important goal of AI is to enable computers to simulate the data processing of the human mind and ultimately improve upon it in terms of speed and accuracy. As noted above, the USPTO recently sought public comment on a variety of questions related to AI, including whether there are any patent eligibility considerations unique to AI inventions and whether current laws and regulations need to be revised to address AI's contribution to the conception of an invention and AI's potential ownership of an invention. Participants in the U.S. biopharma industry will want to monitor these and other legal developments to inform patent strategy related to AI inventions.
2. Where is the value?
Machine learning begins with a training dataset, such as a collection of genomic sequence data, that is fed into an algorithm to train the model. The dataset is the standard against which the algorithms are trained and has undeniable value. Once machine learning algorithms discover patterns in the data, they then apply the training to analyze new data. Until recently, machine learning algorithms based on neural networks relied on researchers to process data before feeding it into the computational models. However, deep learning is a critical advance in machine learning, in which computers independently extract meaningful information from the data and create a predictive tool based on patterns identified in the data.
These data and algorithms create a number of fundamental IP issues. For example, although there may be value in the data used to train a deep neural network, a dataset may not be patentable (and may even be open source). Furthermore, an algorithm that is constantly changing may not be protected if it differs from its description in a patent application. Another consideration is whether the use of complex algorithms will be characterized as mental steps and thus unpatentable—even if a human would be unable to perform them with reasonable accuracy in a reasonable amount of time.
3. What is the best way to protect the IP?
Although patents have historically been considered the best protection for pharmaceutical innovations, they may not cover important aspects of AI, such as AI training sets, source code, or algorithms. Pharmaceutical companies should consider a diversified approach to IP protection that includes not just patents, but also copyrights and trade secrets.
Copyright protection may be available for source code and datasets, but notably there currently is a human authorship requirement for copyrighted works. Thus, human-authored source code and datasets for use in AI applications would be copyrightable (assuming all requirements for copyrightability are met) under the current framework, but such works authored entirely by AI would not. To what extent a human needs to be involved in order to be considered an "author" of a copyrighted work remains unresolved.
Trade secret protection may be available for certain aspects of AI technology—a form of protection that has become potentially more appealing in view of the confusion surrounding patent eligibility. Trade secrets are well-suited to technologies that are not easy to reverse engineer, technologies in a rapidly changing field, and technologies that are difficult to describe—all characteristics that, broadly speaking, are applicable to AI. However, safeguards are required to reasonably maintain the secrecy of a trade secret, which may hinder further development within the company or may make the company less willing to share secret information with third parties and collaborators. In addition, it is unclear how the law will deal with a trade secret that is created by AI—how will that trade secret be identified and its secrecy reasonably maintained? And does the company that owns the AI own the trade secret?
What's Next?
AI is seeing widespread adoption in the biopharmaceutical industry. It is being harnessed to accelerate each step of the R&D process and to improve diagnostics, provide predictive analytics, and fulfill the promise of personalized medicine. The legal landscape will continue to mature, likely with new USPTO guidance—possibly legislation—and, inevitably, litigation. The period for the USPTO's request for comments on patenting AI inventions has now closed, and the USPTO will likely release additional guidance in the new year. There are also bipartisan congressional efforts to address the ongoing confusion and controversy surrounding patent eligibility. A statement released by Senators Tillis and Coons indicates that the effort would address "studies showing that investors familiar with the current lack of clarity invest less in critical research and development in areas like medical diagnostics and artificial intelligence." And, of course, actual conflicts relating to valuable IP will inevitably result in litigation, so various legal theories will be tested and resolved in court. The biopharmaceutical industry should continue to watch for developments in the law, including in the USPTO, Congress, and the courts, so it can continue to innovate while navigating IP laws that are evolving to respond to the rapid pace of development of AI.
Three Key Takeaways
- AI has the potential to transform the R&D in the biopharmaceutical industry, but has also disrupted traditional concepts of IP law, leaving many open legal questions.
- In view of these open questions and in order to maximize protection of valuable AI training sets, source code, and algorithms, a biopharmaceutical innovator should consider a diversified approach to IP protection that includes patents, copyrights, and trade secrets.
- A biopharmaceutical innovator should continue to watch for developments in the law, including in the USPTO, Congress, and the courts, so it can continue to innovate while navigating IP laws that are evolving to respond to the rapid pace of development of AI.